The Powers and Perils of Pattern Recognition
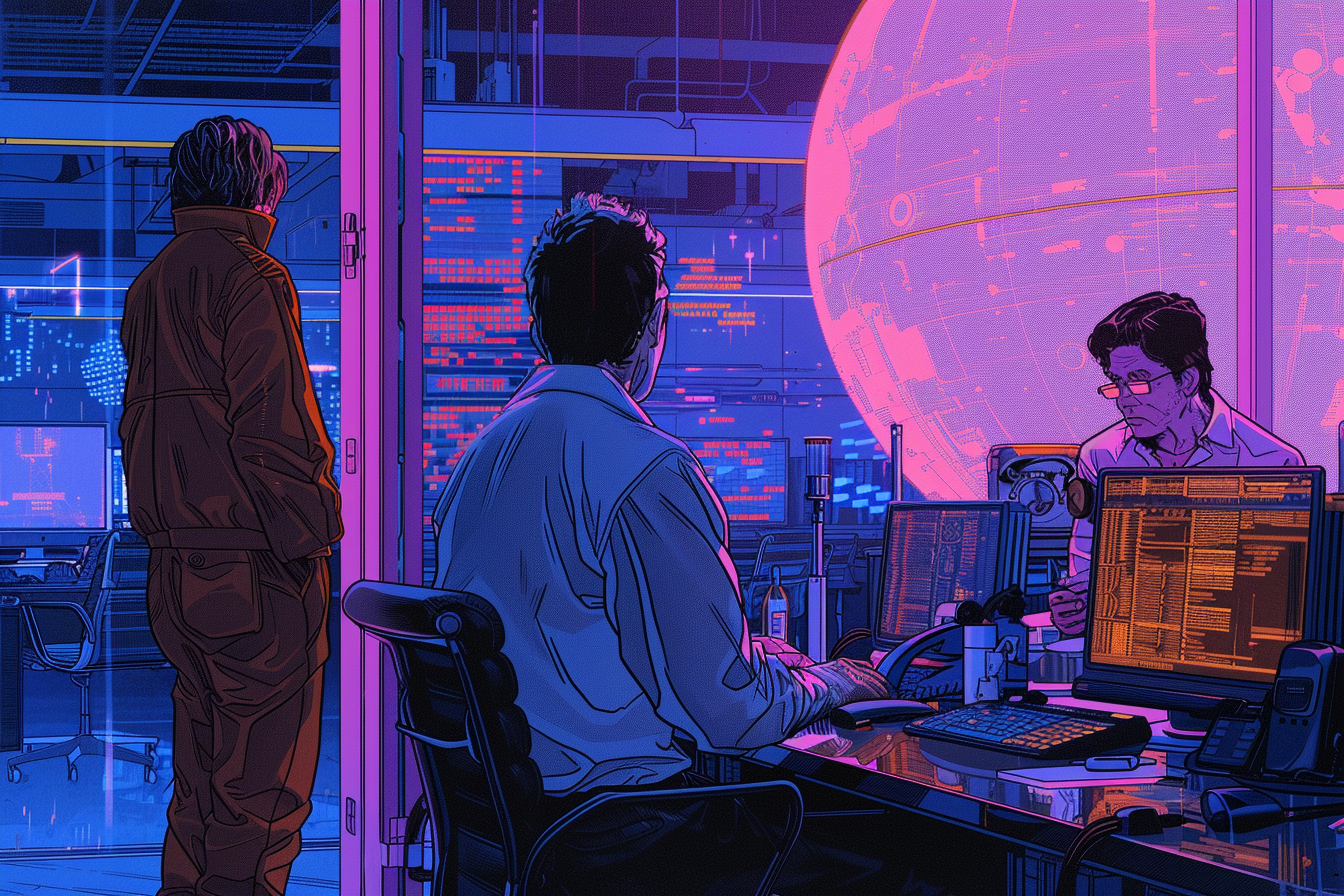
Table of Contents
Pattern recognition is a term that gets tossed around a lot, especially by investors when they’re sizing up early-stage companies. It goes something like this:
"Why did you invest in that company?"
"The founder/company/idea reminds me of..."
I mean, the logic is sound. If you've been investing in early-stage companies for 10 years you've built pattern recognition. Right?
But few stop to ask when does this skill actually help, and when can it mislead us?
Michael J. Mauboussin’s "Pattern Recognition: Opportunities and Limits" paper offers some valuable insights (and IMHO is a must-read from the Mauboussin canon). Here are some insights I gleaned from the paper.
When Does Patter Recognition Help Us?
Pattern recognition works beautifully in stable environments where the cause-and-effect relationships are clear. Think about chess masters. They can spot optimal moves almost instantly because they recognise patterns on the board. The game's consistent rules and immediate feedback let them anticipate several moves ahead with impressive accuracy.
The key to good pattern recognition is feedback. In weather forecasting, experts use advanced models to predict conditions and then immediately see how their predictions pan out. This constant loop of prediction and feedback helps them fine-tune their accuracy. The same goes for athletes—they get instant feedback on their performance, which helps them improve continuously.
In the Mauboussin paper, he references Robin Hogarth, a cognitive psychologist and his view on how environment impacts the power of intuition. He talks about "kind" versus "wicked" environments. In kind environments, the outcomes clearly reflect the quality of decisions, and feedback is abundant. This kind of feedback loop is essential for developing strong pattern-recognition skills.
When Does Pattern Recognition Fail Us?
Here's where things get tricky. Pattern recognition doesn't work so well in unstable environments where the cause-and-effect relationships are murky or constantly shifting. Take the stock market. Its volatility and complexity often lead investors to see patterns that aren't really there, resulting in bad predictions.
In wicked environments, outcomes don’t reliably indicate the quality of decisions, and feedback can be misleading. Complex adaptive systems like ecosystems or economic markets are filled with interacting components, making it tough to discern clear patterns.
Experience alone isn't enough to make someone an expert. Gregory Northcraft highlights that true experts have predictive models that go beyond just experience. Cognitive biases, like the representativeness heuristic, can lead to overconfidence and flawed judgments. We tend to see what we want to see, not what's actually there.
Analogies can also trip us up. Using analogies without understanding their causal relevance can be misleading. Effective pattern recognition needs analogies that aren't just surface-level similar but also causally connected to the problem at hand.
Trusting your intuition isn’t always straightforward. You need to be careful about the environment you’re making decisions in. In stable, feedback-rich settings, your intuition can be a powerful ally. But in dynamic, complex systems, you need to be more cautious. This is why “pattern recognition” in early-stage investing is often overstated. The environments are too dynamic and unpredictable for reliable patterns to emerge. Understanding these nuances can help you leverage the power of pattern recognition while avoiding its pitfalls.
Alan Tsen Newsletter
Join the newsletter to receive the latest updates in your inbox.